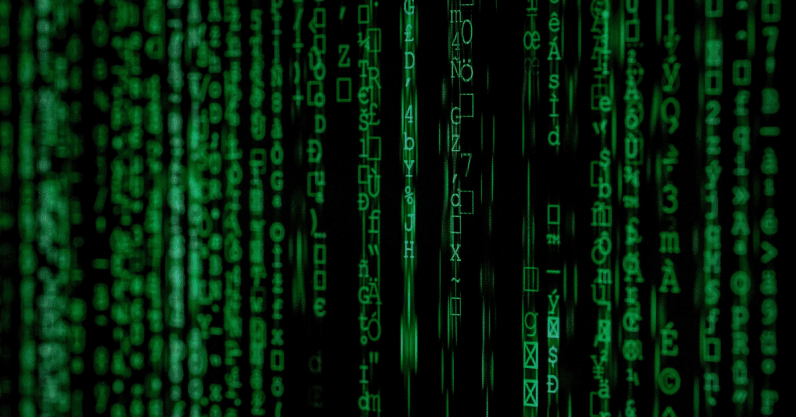
If you’ve ever used a neural network to solve a complex problem, you know they can be enormous in size, containing millions of parameters. For instance, the famous BERT model has about ~110 million. To illustrate the point, this is the number of parameters for the most common architectures in (natural language processing) NLP, as summarized in the recent State of AI Report 2020 by Nathan Benaich and Ian Hogarth. You can see this below: In Kaggle competitions, the winner models are often ensembles, composed of several predictors. Although they can beat simple models by a large margin in terms of…
This story continues at The Next Web
from The Next Web https://ift.tt/3miEaGu
Comments
Post a Comment